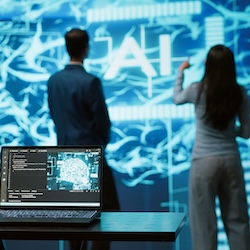
Artificial intelligence (AI) is rapidly transforming industries, influencing everything from business decisions to everyday life. Ensuring these AI systems are trustworthy, transparent, and reliable has become essential. This is where AI Observability steps in—providing systematic monitoring, analysis, and visualization of AI models throughout their lifecycle, ensuring they function as intended.
Understanding AI Observability
AI Observability involves comprehensive monitoring of AI models’ internal states, inputs, outputs, and performance over time. By providing deep insights into model behavior, observability enhances trust among users and stakeholders, especially critical in sectors like healthcare, finance, and autonomous driving. It also helps organizations optimize performance and maintain model reliability.
Transparency Builds Trust
Transparency is essential for understanding AI decisions. AI models are often seen as mysterious “black boxes,” creating uncertainty around how they arrive at specific outcomes. Observability addresses this issue by clearly revealing decision-making processes. For instance, in healthcare, understanding why a diagnosis was suggested by an AI model builds trust between medical professionals and patients, enhancing acceptance and confidence in AI-driven care.
Ethical Compliance and Regulatory Alignment
Regulatory frameworks such as the European Union’s Artificial Intelligence Act emphasize ethical and responsible AI use. Observability helps organizations comply with such regulations by tracking model decisions and ensuring adherence to ethical guidelines. By providing clear accountability, AI observability promotes responsible AI governance and reduces regulatory risks.
Performance and Reliability Management
AI systems, particularly those utilizing deep learning, require extensive computational resources. Observability identifies performance bottlenecks, resource utilization inefficiencies, and optimization opportunities, significantly improving performance while reducing costs. Metrics like stability, latency, and model drift are continuously monitored, ensuring AI models maintain accuracy and reliability over time.
Data Observability: Ensuring Data Integrity
Data quality is central to ethical and effective AI systems. Data observability, an essential component of AI observability, focuses on maintaining data integrity, proactively identifying biases, and ensuring high-quality data inputs. Detecting and mitigating biases such as selection, confirmation, and labeling biases ensures fairer and more equitable AI outcomes.
Observability Across AI Architectures
AI observability operates across multiple layers of AI architecture:
- Orchestration Layer: Monitors workflows, resource management, and operational efficiency.
- Semantic Layer: Ensures accurate data interpretation and retrieval, vital for meaningful insights.
- Model Layer: Directly tracks performance metrics, service-level indicators like latency, availability, and error rates.
Explainable AI (XAI): Making AI Understandable
Explainable AI techniques, such as Local Interpretable Model-agnostic Explanations (LIME) and SHapley Additive Explanations (SHAP), offer transparency by clarifying why models produce certain outcomes. Programs like DARPA’s XAI initiative further highlight the balance between interpretability and maintaining high performance, driving innovation in transparent AI modeling.
Mitigating Bias for Equitable AI
Bias in AI models threatens fairness and trust. Observability helps organizations detect biases through statistical analysis and visualization methods, such as disparity heatmaps. Addressing selection bias, framing bias, and labeling bias ensures equitable AI outcomes, essential in applications like hiring or loan approvals.
Essential Metrics for AI Observability
Key metrics monitored through AI observability include:
- Stability: The success rate of model predictions.
- Latency: The response time of models from request to output.
- Model Drift: Performance changes due to shifting real-world data.
- Data Drift: Changes in data characteristics affecting model accuracy.
Real-World AI Observability in Action
Organizations, including DARPA and Splunk, demonstrate real-world applications of AI observability. For instance, Splunk integrates observability to enhance predictive analytics, monitor system health, and provide real-time feedback. These capabilities help organizations proactively resolve potential issues, optimize system efficiency, and maintain robust AI systems.
Challenges and Future Opportunities
AI observability faces several challenges:
- Complexity and Scale: Managing extensive data volumes and complex models.
- Balancing Transparency with Performance: Ensuring explainability without sacrificing accuracy.
- Generative AI Reliability: Addressing “hallucinations,” or inaccurate outputs, common in generative AI.
Looking ahead, future advancements may include greater automation, enhanced explainability, and closer integration of generative AI and observability platforms. Continued innovation will align AI systems more closely with strategic business outcomes, ensuring that observability remains integral to responsible AI deployment.
Conclusion
AI Observability is not merely beneficial—it is essential for deploying trustworthy, transparent, and ethical AI systems. By enabling organizations to manage biases, optimize performance, and ensure regulatory compliance, observability reinforces AI’s role as a trusted, reliable partner in decision-making processes. As AI continues evolving, robust observability practices will be crucial to harnessing AI’s full potential while addressing its inherent risks.
About the Author
Rambabu Bandam is a seasoned technology leader with over 18 years of experience in the industry, specializing in AI, cloud computing, big data, and analytics. He currently serves as Director of Engineering at Nike, where he leads teams focused on building large-scale, real-time data platforms and AI-powered analytics solutions. Rambabu has a strong background in cloud architecture, data governance, and DevOps, and has been instrumental in optimizing enterprise data ecosystems across multiple Fortune 500 companies. His technical expertise spans AWS, Databricks, Kafka, and machine learning, driving innovation, scalability, and data-driven decision-making. Follow Rambabu on LinkedIn.
Disclaimer: The author is completely responsible for the content of this article. The opinions expressed are their own and do not represent IEEE’s position nor that of the Computer Society nor its Leadership.