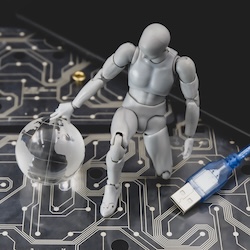
Artificial intelligence (AI) and machine learning (ML) are among today’s most transformative technologies. Yet, according to Gartner Research, only nine percent of technology leaders report using generative AI (GenAI) projects in production, as opposed to 83 percent with projects in the pilot or active assessment phase. These numbers underscore the alarmingly prevalent issue of AI projects stagnating in experimental stages without transitioning to scalable, impactful business solutions. This is known as a proof-of-concept (PoC) trap. Chief technology officers (CTOs) and chief product officers (CPOs) can ensure that AI initiatives transition to scalable, impactful business solutions instead of falling into the PoC trap by adopting a proactive approach to avoid common pitfalls for ML projects.
Data-Related Challenges and Building Strong Platforms
Data is the lifeblood of ML model development. Yet, the overwhelming majority of failures emanate from data shortcomings. Poor-quality data, substandard data governance, and data that lack real-world representation are persistent issues for most organizations and lead to suboptimal models. Building an accurate and reliable ML model depends on high-quality training data, as a lack of clean, well-labeled, and thorough data remains one of the most frequent reasons behind PoC failures. Poor real-world representation creates biases, resulting in discriminatory predictions from a flawed model. This is observed in both classical AI (such as models for hiring practices or loan approvals) and GenAI (such as content generation). Without strong data governance, organizations risk falling out of regulatory compliance and compromising privacy.
Organizations with successful implementations employ a robust data strategy with sufficient data availability, quality, and governance. Scalable platforms capable of handling data volume, velocity, and variety open new potential for AI innovation. It’s imperative for organizations to assess whether this data is sufficient to adapt to real-world conditions. Smaller datasets may introduce biases and fail to generalize and perform in production. Many PoCs fail due to underestimation of the significance of clean, structured data in the development of ML models. By investing in data infrastructure from the outset—such as building data platforms, establishing extract transform load (ETL) pipelines, and implementing strict data governance—organizations can ensure high-quality data that is aligned with strategic objectives and regulatory requirements.
ML Model Development
Developing robust models with strong generalization capabilities and the ability to meet nuanced, production-grade requirements is pivotal to avoiding the PoC trap. A major obstacle in this area is overfitting or failing to maintain accuracy when transitioning from training to real-world data. PoC environments with limited datasets or a lack of real-world use case representation exacerbate the overfitting issue. Most advanced ML models are black-box systems, complicating the abilities of developers or other stakeholders to comprehend the rationale behind specific outputs. Such lack of transparency can create significant legal and bias-related issues, specifically in industries where the rationale behind a model’s output must be explainable.
GenAI applications are becoming common targets for data poisoning and prompt injection attacks, in which malicious actors manipulate inputs and cause the model to generate harmful or misleading content. This practice is especially dangerous for public-facing chatbots, as incorrect or inappropriate content can reduce trust and damage a brand’s reputation.
Professionals can mitigate the risk of overfitting by applying regularization techniques, employing cross-validation, and opting for more interpretable models. Adopting simpler and more interpretable models during the PoC phase can offer more generalization before upscaling to more complex model architecture for production. For instance, developers can use local interpretable model-agnostic explanations (LIME) or SHapley Additive exPlanations (SHAP) to explain complex black-box models. These techniques provide insight into the specific features that influence a given output. Additionally, adversarial training and prompt guardrails against adversarial attacks will improve resiliency, while robust content moderation ensures safe operability in public-facing environments.
Challenges in Production Deployment and Ethical Issues
When transitioning from PoC to production, organizations face significant challenges in areas including performance, scalability, cost, and infrastructure limitations. Probabilistic outputs in GenAI projects often suffer from insufficient error handling and customization issues, causing considerable risk. Successful organizations address deployment and scalability challenges via the adoption of MLOps practices. Automation of the ML lifecycle enables a smoother transition from PoC to production, while available techniques, including sharding, quantization, distilling, and caching, allow users to optimize computation and memory infrastructure, helping reduce cost and enhance performance.
Because many PoCs use abstraction frameworks that are not designed to scale effectively and handle customization needs in production environments, it is essential for developers to treat these frameworks as prototyping tools. Critical evaluation of such frameworks, followed by the development of custom components to optimize performance and scalability, is imperative for a successful progression into the production phase.
Legal and ethical challenges can stall ML model deployment. This is mainly due to the utilization of large black-box models with vulnerabilities to amplify bias, particularly when the models are trained using data that reflects outdated historical inequalities or prejudices. Uncertainty surrounding data provenance or ownership, use of proprietary or third-party datasets, and intellectual property add additional hurdles. GenAI-specific endeavors mean more legal challenges pertaining to ownership of AI-generated content, plus copyright and data privacy laws.
The adoption of ethical frameworks emphasizing fairness, accountability, and transparency (often expressed as FAT) combined with the implementation of regular audits for bias, data governance structure, and strict content moderation systems increases compliance with legal standards. To ease this process, development teams can work with legal counsel and additional experts from the outset of each project to develop clear guidelines and safeguards against legal disputes and ethical risks.
Legal rulings reflect the reality that organizations are responsible and liable for the output of AI systems. In a 2022 case, a customer sought damages after a chatbot gave inaccurate information regarding a discount that wasn’t available. The organization argued that the chatbot was a “separate legal entity responsible for its own actions.” The court ruled in favor of the customer, stating it “should be obvious” that the company is responsible for all information on its website.
Organizational Challenges
The allure of technical innovation can be so tempting that organizations neglect the strategic business issues that ML models are used to solve. Too often, the result is a technologically impressive PoC that falls short of demonstrating financial value, making justification of further investment challenging. This failure is frequently caused by a misalignment between project objectives and tangible business goals stemming from a lack of cross-functional collaboration, leading to frustrating projects that struggle and never surpass the PoC phase.
It’s imperative for organizations to focus on cooperation and cross-collaboration from the outset to implement successful projects. Addressing and correcting technical issues require proficiency, expertise, and experience—yet it is easier than fixing damaged relationships or dysfunctional professional cultures. Fostering all-inclusive teams of data scientists, engineers, business leaders, legal, sales, and domain experts creates a collaborative environment where everyone takes ownership of the project. Incremental growth and development are critical to the progressive expansion of project scope and scale, increasing the chances of long-term organizational success.
ML and AI initiatives can transform organizations and permanently improve customer interactions. Creating technological advances cannot come at the expense of addressing actionable business objectives. By structuring initiatives around robust data, strong model development, legal and ethical compliance, and inter-department collaboration, organizations can ensure a successful transition of ML products from the PoC phase to production.
About the Author
Alok Abhishek is a product management leader with over 15 years of experience in AI, data management, and cloud technologies. Alok received the 2024 Globee Award for Artificial Intelligence Achiever of the Year in recognition of his contributions to the open-source AI community. He holds an MBA, a graduate certificate in data science from the University of Southern California, a bachelor’s degree in computer science from Manipal Institute of Technology, and multiple industry certifications. Connect with Alok on LinkedIn.
Disclaimer: The author is completely responsible for the content of this article. The opinions expressed are their own and do not represent IEEE’s position nor that of the Computer Society nor its Leadership.