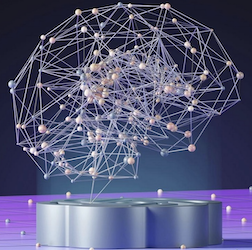
Artificial Intelligence (AI) has rapidly become a cornerstone of decision-making across critical systems, from healthcare and finance to judicial processes and public safety. According to a poll conducted by Thomson Reuters, 91% of C-suite executives say that they have plans to implement AI tools in some way, shape, or form within the next 18 months [1]. While AI’s computational power and ability to analyze vast datasets offer unparalleled efficiency, the ethical implications of AI-driven decisions are increasingly under scrutiny. The integration of artificial intelligence into critical decision-making systems has sparked a revolution in how organizations approach complex ethical challenges.
To put things into perspective, take for example the healthcare industry. As of 2024, 43% of healthcare leaders report leveraging AI for in-hospital patient monitoring systems, while 85% are investing in or planning to invest in generative AI technologies in the next 3 years [2]. It is estimated that by the end of 2025, nearly 90% of hospitals will utilize AI-powered technologies for early diagnosis and remote patient monitoring [3]. However, this widespread adoption has brought to the forefront a crucial challenge: ensuring these systems make fair and transparent decisions that can be understood and trusted by all stakeholders.
The Rise of Explainable AI
Explainable AI (XAI) has emerged as a cornerstone in addressing the “black box” nature of complex AI systems. Unlike traditional AI models that operate as opaque decision-makers, XAI techniques provide insights into how and why specific decisions are made. This transparency has become increasingly critical as AI systems are deployed in sensitive areas such as loan approvals, medical diagnoses, and employment screening.
For example, a novel method proposed in a research titled “Enhancing Financial Risk Management with Federated AI” The authors propose a solution that combines Federated Learning (FL) and Explainable AI (XAI), enabling institutions to collaboratively train models without sharing sensitive data, thereby protecting privacy while ensuring model transparency and interpretability [4]. This method addresses the challenges financial institutions face in detecting fraudulent transactions, particularly the rarity of fraud cases leading to imbalanced datasets, strict privacy regulations limiting data sharing, and the necessity for transparency to maintain user trust.
According to a report by McKinsey, organizations prioritizing digital trust, including enhancing AI explicability through explainable AI, are likely to experience annual revenue and EBIT (earnings before income and taxes) growth rates of 10% or more [5]. These improvements stem from the ability to provide clear, understandable explanations for AI-driven decisions to stakeholders, consumers, as well as investors.
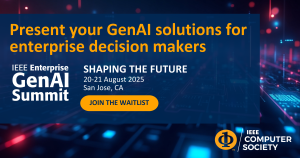
Core XAI Techniques and Their Applications
As AI systems grow increasingly sophisticated, the need for transparency has also driven innovation in interpretability techniques. Recent advances in explainable AI have moved beyond simple feature importance rankings to sophisticated methods that can unpack the decision-making process of even the most complex models. These techniques strike a delicate balance between preserving model complexity and providing meaningful explanations that resonate with both technical and non-technical stakeholders. Several key techniques have emerged as standards in making AI systems more explainable. Following are some of the key XAI techniques that exists today:
- LIME (Local Interpretable Model-agnostic Explanations) has revolutionized how we understand individual AI decisions. By creating simplified, interpretable models that approximate complex AI decisions locally, LIME allows stakeholders to understand which factors most influenced specific outcomes. In short, LIME creates local approximations of complex models using simpler, interpretable models [6]. For instance, in healthcare, LIME helps doctors understand why an AI system flags certain patients as high-risk, enabling more informed clinical decisions. The following figure shows the high-level LIME workflow.
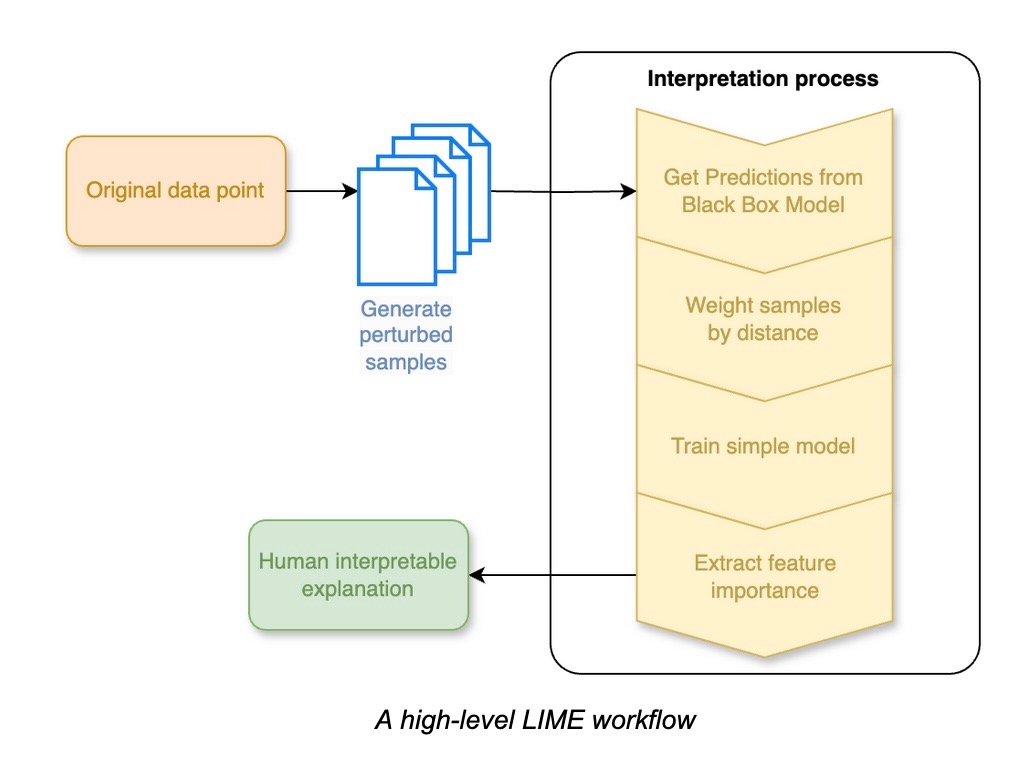
- SHAP (SHapley Additive exPlanations) values provide a game-theory approach to feature importance, offering a unified framework for interpreting model predictions. This technique has proven particularly valuable in financial services, where regulations require clear justification for lending decisions. SHAP uses game theory to calculate each feature’s contribution to the final prediction. The following figure explains a high-level SHAP workflow [7].
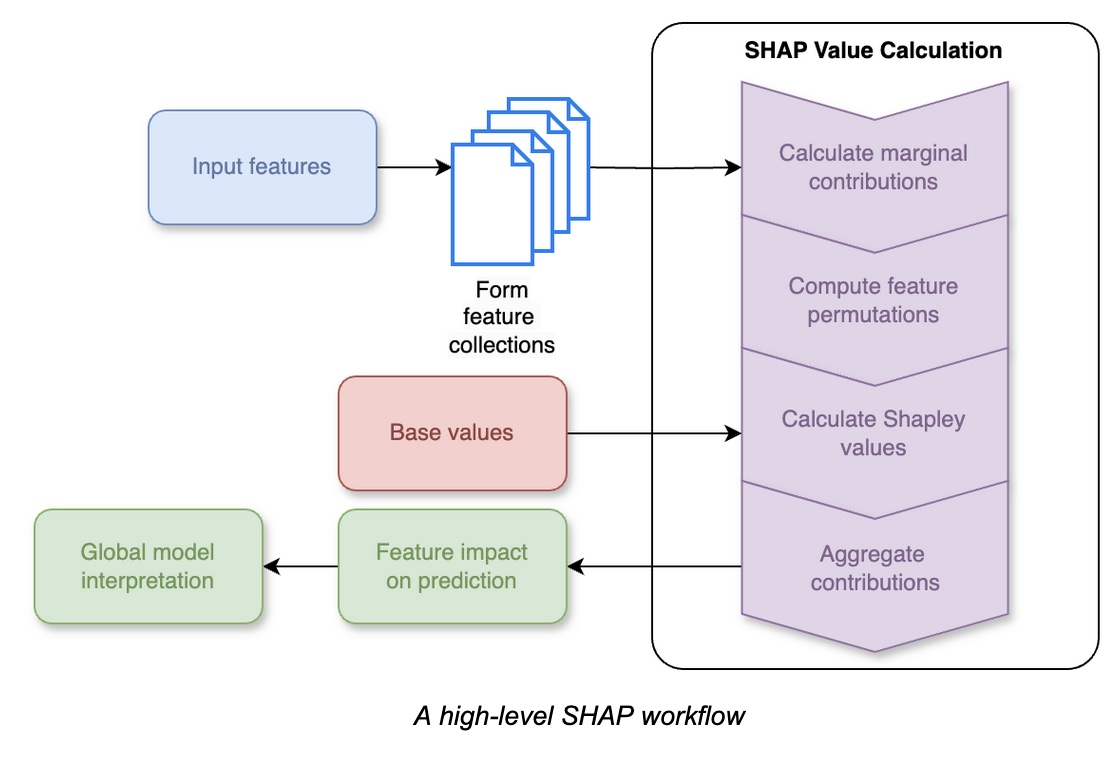
- Counterfactual explanations: Complementing LIME and SHAP, counterfactual explanations represent a different yet powerful approach to AI interpretability. While LIME and SHAP help understand why a decision was made, counterfactuals answer the crucial question “What would need to be different to get a different result?” For example, in a loan application scenario, instead of just explaining that low credit score and high debt ratio led to rejection, a counterfactual explanation might specify that “If your credit score was 50 points higher and your debt-to-income ratio was 5% lower, your loan would have been approved.” This actionable insight helps users understand not just the ‘why’ behind decisions, but also the ‘how’ of achieving different outcomes. In healthcare settings, counterfactuals might show doctors which specific changes in patient metrics would move a patient from high-risk to low-risk categories, enabling more targeted interventions.
Building Ethical Frameworks around XAI
The implementation of XAI requires more than just technical solutions; it demands a comprehensive ethical framework that guides its deployment. Organizations leading in this space have developed structured approaches that combine technical capabilities with ethical considerations, these include:
- Fairness Auditing: Organizations must implement systematic evaluation processes to ensure AI decisions remain equitable across all demographic groups. This involves regular statistical analysis of outcomes across different populations, tracking metrics like false positive/negative rates and decision distribution patterns. For example, in hiring systems, this might involve monthly reviews of recommendation rates across gender, age, and ethnic groups, with automated alerts for any statistically significant disparities. Modern fairness auditing also incorporates intersectional analysis, examining how multiple demographic factors interact in AI decision-making, and employs bias-detection algorithms to proactively identify potential discrimination before it impacts users.
- Stakeholder Engagement: Successful AI implementation requires more than technical excellence; it demands active participation from those affected by its decisions. This means establishing formal feedback channels, advisory boards, and regular community consultations. Organizations should conduct periodic workshops with end-users, industry experts, and affected communities to gather insights about the real-world impact of AI decisions. For instance, in healthcare AI systems, this might involve regular meetings with patients, healthcare providers, and medical ethicists to understand how AI recommendations influence treatment decisions and patient outcomes. These engagements should be structured to ensure representation from diverse perspectives and experiences.
- Transparency Protocols: Beyond basic documentation, organizations need comprehensive transparency frameworks that evolve with their AI systems. This includes maintaining detailed model cards that document training data sources, performance metrics, and known limitations. Organizations should establish clear version control practices for AI models, maintain logs of all system updates, and provide accessible explanations of decision-making processes tailored to different stakeholder groups. For example, a lending institution might maintain technical documentation for regulators, simplified explanations for loan officers, and clear, actionable information for loan applicants about how the AI influences lending decisions.
- Appeal Mechanisms: Effective appeal processes must be more than just a formality; they should be accessible, timely, and genuinely capable of affecting outcomes. This involves creating multi-tiered review systems where challenges can be escalated based on complexity or impact. Organizations should establish clear timelines for appeal responses, provide multiple channels for submitting appeals (digital and non-digital), and ensure human experts are available to review complex cases. The appeal system should also feed back into the broader AI governance framework, with patterns in appeals used to identify potential systemic issues in the AI decision-making process. For instance, a credit scoring system might offer both quick automated reviews for clear-cut cases and detailed human reviews for more complex situations, with all appeals documented to improve future model iterations.
Conclusion
Despite significant progress, several challenges remain in implementing XAI effectively. The balance between model complexity and explainability continues to be a central challenge, as more sophisticated AI systems often provide better performance but are harder to explain. Additionally, ensuring explanations are meaningful to different stakeholders – from technical experts to affected individuals – requires careful consideration of communication strategies. Looking ahead, emerging trends suggest several promising directions for XAI such as:
- Interactive Explanations: Which deals with the development of dynamic interfaces that allow users to explore AI decisions at their own pace and level of technical understanding.
- Customized Explanation Frameworks: Developing tools that adapt explanations based on the stakeholder’s role, knowledge level, and specific needs.
- Automated Ethical Compliance: Building systems that continuously monitor and adjust AI decisions to ensure adherence to ethical guidelines and regulatory requirements.
As AI continues to penetrate deeper into critical decision-making systems, the role of XAI in ensuring ethical and fair outcomes becomes increasingly vital. Organizations must view XAI not as a technical add-on but as a fundamental component of their AI strategy. This approach requires investment in both technical capabilities and organizational processes that support transparent, accountable AI systems. The future of ethical AI decision-making lies in creating systems that are not only powerful and accurate but also transparent and fair. By embracing XAI techniques and building robust ethical frameworks around them, organizations can harness the full potential of AI while maintaining the trust and confidence of all stakeholders involved.
As we move forward, the success of AI in critical systems will be measured not just by its technical performance, but by its ability to make decisions that are explainable, fair, and aligned with human values. The continued evolution of XAI techniques and ethical frameworks will play a critical role in achieving this vision, ensuring that AI remains a force for positive change in society.
References:
[1] https://www.thomsonreuters.com/en-us/posts/corporates/future-of-professionals-c-suite-survey-2024/
[2] https://www.ottehr.com/post/what-percentage-of-healthcare-organizations-use-ai
[3] https://www.dialoghealth.com/post/ai-healthcare-statistics
[4] Dhanawat, V., Shinde, V., Karande, V., & Singhal, K. (2024). Enhancing Financial Risk Management with Federated AI. Preprints. https://doi.org/10.20944/preprints202411.2087.v1
[5] https://www.mckinsey.com/capabilities/quantumblack/our-insights/why-businesses-need-explainable-ai-and-how-to-deliver-it
[6] Scott M. Lundberg and Su-In Lee. 2017. A unified approach to interpreting model predictions. In Proceedings of the 31st International Conference on Neural Information Processing Systems (NIPS’17). https://dl.acm.org/doi/10.5555/3295222.3295230
[7] Marco Tulio Ribeiro, Sameer Singh, and Carlos Guestrin. 2016. “Why Should I Trust You?”: Explaining the Predictions of Any Classifier. In Proceedings of the 22nd ACM SIGKDD International Conference on Knowledge Discovery and Data Mining (KDD ’16). https://doi.org/10.1145/2939672.2939778
About the Author
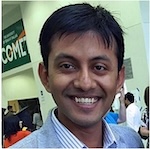
Vineet Dhanawat is a Software Engineer at Meta Platforms Inc.. He received his Master’s degree in Computer Science from the University of Texas at Dallas, United States, in 2015 and his Bachelor’s degree in Computer Engineering from Birla Institute of Technology and Science, Pilani, India, in 2011. Over the past 14 years, he has worked for several big tech companies, where he has been entrusted with leading teams and tackling complex challenges head-on. He has held leadership roles in various organizations, driving innovation and growth through strategic technology implementations. His areas of interest include Machine Learning, Artificial Intelligence, and Integrity. Connect with Vineet Dhanawat on LinkedIn.
Disclaimer: The author is completely responsible for the content of this article. The opinions expressed are their own and do not represent IEEE’s position nor that of the Computer Society nor its Leadership.